- AI in Lab Coat
- Posts
- Biases in AI Medical Devices Surge as the FDA Tries to Keep Up - Are We Ignoring the Inequities?
Biases in AI Medical Devices Surge as the FDA Tries to Keep Up - Are We Ignoring the Inequities?
In our eagerness for AI-driven healthcare, are we foregoing the need for stringent testing?

In January of this year, the FDA approved DermaSensor, the first artificial intelligence (AI)-powered device for primary care physicians to detect skin cancer. This major breakthrough showcases the potential of AI technologies in healthcare, but we rarely discuss the risks.
Biases embedded in AI algorithms often reflect our societal shortcomings. In my experience with AI-enabled health projects over the past few years, I find that these biases show up in two ways.
First, the health data used to train AI models is often unbalanced, representing only certain demographic groups. For example, in my previous article on AI in space medicine, I highlighted the usefulness of AI health assistants for astronauts, given the critical need for maintaining health in space.
However, since only 11% of astronauts have been women, the training data for these algorithms has been overwhelmingly collected from men. What risks do female astronauts face when relying on AI trained predominantly on male data?
Gender, ethnic, and socioeconomic biases are common in training data. We need to be aware of these biases when developing an AI model for use in medical devices.
Second, these biased algorithms are then tested on similarly biased clinical groups, which predictably yield good results but are not truly representative of the broader population. This cycle perpetuates inequities, potentially giving a false sense of efficacy and safety.
A Case Study: DermaSensor — Balancing Innovation with Caution
I want to start by making my stance clear. I am a major advocate for AI in health and biotech research. I believe AI can enhance diagnostic accuracy, reduce healthcare costs, and accelerate medical innovation.
My hope is to raise awareness and ensure that this rapidly advancing field does not escape our common judgement — that this technology is made to benefit everyone fairly.
The approval of DermaSensor as a case study is a great example of AI-medical innovation. But it also clearly demonstrates why we should be more vigilant than ever. This historical authorization now sets the regulatory benchmark for how other AI-powered devices will be evaluated.
Why DermaSensor breaks the status quo
The significance of DermaSensor is that it allows primary care physicians (PCPs) to assess skin cancer, a task traditionally handled by dermatologists. This newfound accessibility broadens the scope for non-specialists to offer services previously limited to specialists, setting DermaSensor apart from earlier AI-supported skin cancer devices.
Giving access to initial assessments by your PCP alleviates the current burden dermatologists have with the backlog of cases. This is especially important as the prevalence of skin cancer is on the rise.
In the United States, more than 9,500 people are diagnosed with skin cancer every day, and every hour, 2 people die from this disease. 1 in 5 Americans will develop skin cancer by the age of 70, with more people diagnosed with skin cancer than all other cancers combined.
The issue is that finding or getting a referral to a dermatologist isn’t straightforward, leading to delays or preventing some people from seeing one altogether. This is even more difficult in rural areas where dermatologists are scarce.
Photo by Bermix Studio on Unsplash
One study revealed that 51.8% of people avoided or delayed dermatology care. With 42.9% citing financial and insurance challenges, 33.9% had logistical issues (long-wait times, transportation, finding childcare, etc.), and 40% outright have limited access to a dermatologist.
With tools like DermaSensor, we’re not just advancing technology; we’re redefining the boundaries of who can deliver critical healthcare services and ensuring quality care is within reach for everyone.
Diagnosing skin conditions primarily depends on visual assessment. Differentiating between benign and suspicious lesions, which dictates the need for further tests and treatments, relies heavily on a dermatologist’s clinical expertise honed over years of experience.
For non-specialists like PCPs, differentiating skin cancer from other conditions can be challenging without this developed skill. However, the visual nature of diagnosis means images of lesions can be used in AI and machine learning (ML) to translate that expertise into standardized practice.
Training AI on images to find patterns is a powerful process. It’s also the reason why we’ve seen the most AI medical advances in radiology. However, the effectiveness of an AI algorithm is only as good as the quality of its data inputs. If biases exist in the data, the output will reflect this bias.
Unfortunately, I was not able to find the original dataset used to develop the skin cancer detection model. But a DermaSensor funded study revealed that over 10,000 readings and 2000 lesions were used to train their AI. Without further information, I cannot comment on the demographics and quality of its inputs.
However, we can look at the summary reports that led to the FDA’s decision to authorize the use of DermaSensor: their pivotal study (DERM-SUCCESS), supplemental study (DERM-ASSESS III), and clinical utility study.
Their pivotal clinical study, conducted at 18 locations in the US and 4 in Australia, reveals a clear bias in participants' demographics. Out of a pool of 1005 patients, the top three groups in terms of race categorization were Whites (97.1%), Asians (0.9%), and Black or African Americans (0.7%).
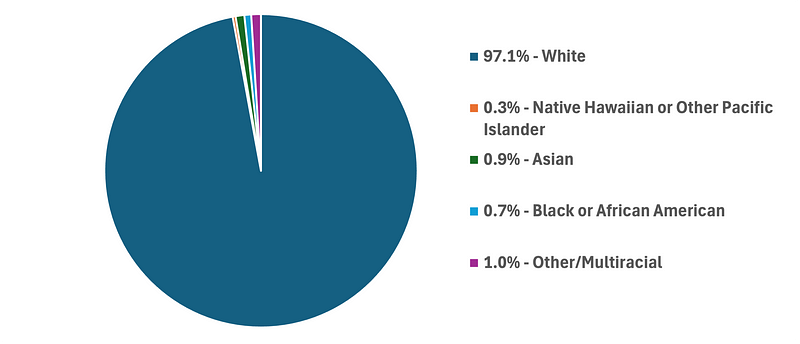
Data from DermaSensor’s DERM-SUCCESS Clinical Study.
Additionally, the study used the Fitzpatrick scale, which classifies skin types based on their response to UV light. Skin types I-IV, which account for lighter skin tones, made up 87.3% of the participants, while skin types V and VI, representing darker skin tones, comprised only 12.7%.
It is important to note that the rates of skin cancer are lower for skin types V and VI. Approximately 2% of Black African Americans in the United States are diagnosed with skin cancer, so at first glance, these rates may seem to represent the tested demographic. However, more recent studies detail many factors that underrepresent the rates of cancer among skin types V and VI.
For example, the 5-year survival rate for black patients is 74%, as opposed to 94% for white patients. Other studies have also noted the underrepresented cancer data in people of color.
Regardless of cancer rates among people with different skin tones, my argument is that an AI-powered medical device should not care whether one’s skin tone is light or dark, but rather, it should be capable of accurately determining whether a person may potentially have cancer or not.
Given all the available data, I don’t believe the developers of DermaSensor intentionally removed or used biased training data. It’s simply a reflection of the current circumstances.
However, this is also the reason why we need to do better. Gathering more diverse data is critical in ensuring that AI technologies serve and represent the full spectrum of our population.
The FDA’s approval process is a benchmark for global standards
DermaSensor’s authorization represents the latest advancement in the growing trend of AI-enabled medical devices receiving regulatory approval in the U.S. and globally. In my opinion, the FDA will play a pivotal role in shaping the regulatory framework of AI-powered medical devices.
Photo by National Cancer Institute on Unsplash
Many global regulatory bodies use the FDA as a benchmark because of its rigorous standards and thorough review processes. The FDA’s approval is often seen as a sign of quality and safety, so other certifiers may fast-track their own approval processes.
This level of authority also comes with significant responsibilities. Currently, the U.S. does not have a healthcare-specific policy for AI use. In fact, the current state of AI regulation in healthcare is fragmented among other federal agencies. Without direct guidance from a unifying policy specific to AI implementation in healthcare, the FDA is left to navigate these challenges on its own.
The FDA’s temporary balance in regulating AI medical devices
It’s not easy. From my perspective, the FDA will soon be at a crossroads: either updating its current evaluation and approval processes or implementing a completely new framework for AI-powered medical devices.
Just remember, the FDA’s regulatory guideline for medical devices was created when most devices were primarily physical equipment. As technology advanced, software was integrated into these devices. Historically, the use of software was mainly for interfacing with the device, with the primary utility still being physical in nature.
As we adopt AI, the utility shifts from hardware to software, meaning the device heavily relies on the software for its function (or AI programming in this context). The FDA recognizes this transition and calls it “Software in a Medical Device” (SiMD).
As of May 2024, there are 882 FDA-approved AI/ML-powered devices. To truly appreciate the challenge the FDA is facing, consider that 84% of these devices have been approved in the last five years. Such rapid authorization rates raise questions about whether current regulations are sufficient to ensure the safety and efficacy of these devices.

Data from FDA.
As AI becomes more integrated into everyday life, there will come a day when medical diagnosis may even be performed by the average citizen. Software integrated into your smartphone or other wearable technology (watch, glasses, headphones, etc.) with medical diagnostic capability will be categorized as “Software as a Medical Device” (SaMD).
The major difference between SiMD and SaMD is the software’s ability to function independently from the hardware.
For example, DermaSensor, although powered by AI software, still depends on its hardware, which uses ‘elastic scattering spectroscopy’ to capture lesion data prior to AI analysis, thus it is termed SiMD.
If their software were a downloadable app for your smartphone, allowing you to take pictures of the lesion for AI analysis, it would be referred to as SaMD.
Beyond regulation: Challenges facing AI in healthcare
Despite the surge in AI technology, the FDA’s approach to regulating these devices is notable for its focus on equity. This ensures that the development of new products meets public health requirements, supported by after-market validation. Reading the FDA’s correspondence letter to DermaSensor, it’s clear they have also identified such shortcomings.
Applying this equity-focused perspective from the pre-market stage onwards is important for the regulation of all AI-enabled devices.
While the FDA takes appropriate steps toward meaningful regulation, it’s clear that other issues beyond the FDA’s reach will soon need our attention. As AI devices become more common in healthcare, I wonder about the loss of human insight.
Photo by National Cancer Institute on Unsplash
For example, the learned skill of identifying skin cancer by dermatologists is acquired over years of practice. Can that intuition be really programmed? If so, is AI capable of generating new insights? Will future innovations be stunted due to a lack of new training data?
In the short term, DermaSensor adds a valuable AI-enabled tool to the primary care toolkit, enhancing the diagnostic capacity of non-specialist physicians. In the long term, its authorization may become a significant milestone in the regulation of AI-enabled medical devices, setting a precedent for how these technologies are integrated into healthcare.
The regulatory framework for allowing new AI-powered medical devices to enter the market must evolve to maintain high safety standards and yield equitable outcomes for all patients.
The real revolution of AI-powered healthcare will come from its integration with human insight, blending technological precision with compassionate care.
Subscribe for more weekly insights into AI health and biotech research.

What content do you want to see more of? |
Reply